This article is written in English but may be of particular interest to French civil servants. If you’ve got troubles understanding English, please email [email protected]
.
Lately, I wondered if it was possible to know about how people working for the French government produce, publish and contribute to open source code. Having a complete picture is nearly impossible, but I could start with something: analysing commits pushed on GitHub by people who used a @*.gouv.fr
email address.
Gaining knowledge about this subject enables a lot of things: knowing where code is published, meeting fellow developers, building a list of commonly used software, establishing a list of open source software the government contributes to and a lot more.
Methodology
I used Gh Archive which provides a compressed log file for every hour since 2015-01-01 with GitHub events’ API. Each log file contains all public events (events for private repositories are not available there) which happened on GitHub during this hour. GitHub provides 20+ event types (opening a pull request, writing a comment on an issue, pushing a commit etc). These logs are quite huge: for example it was 500 GB (compressed) for 2015 and 1.07 TB (compressed) for 2018.
I downloaded and processed these log files from 2015-01-01 until 2019-03-31. The total number of rows was 1,523,732,038 (1.5 billion events). I only kept rows when a commit was pushed (the PushEvent event) and the main author committed with a @*.gouv.fr
e-mail address. This means that we have a row every time someone commits to a public GitHub repository with a @*.gouv.fr
email address between 2015-01-01 and 2019-03-31.
Limits
Getting a list of all commits done by people coding for the French government is incredibly hard, for a number of reasons:
- A majority on contributions are not made on GitHub or are not even available on the Internet;
- Some developers use their personal laptop with Git already configured with their personal email address and don’t switch to their government one when coding at work;
- I know that civil servants don’t always have a
@*.gouv.fr
e-mail address;
- A very few amount of people commit using their
@*.gouv.fr
e-mail address for now, even if it is recommended in the French government open-source contribution policy (it was recently published in February 2018).
With these important limits in mind, let’s look at the data.
Analysis
Data: we have a row every time someone commits to a public GitHub repository with a @*.gouv.fr
e-mail address between 2015-01-01 and 2019-03-31. There is a total of 24,989 commits in the dataset (only).
First, let’s look at the number of commits by month since 2015.
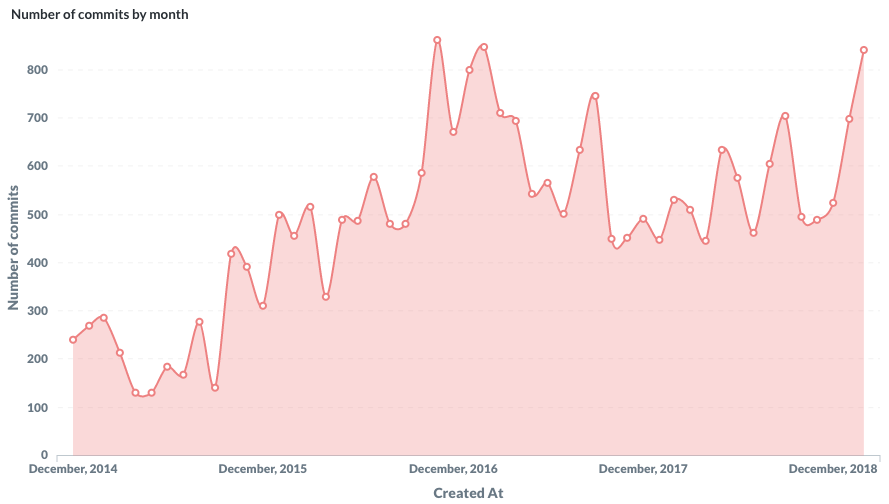
Number of commits by month
Then, let’s look at the number of unique committers by month. I’m glad to notice an upward trend but it’s also very clear that the data is missing a lot of people. According to the data, only 66 people with a @*.gouv.fr
e-mail address did at least a commit in January 2019 which is way less than the reality.
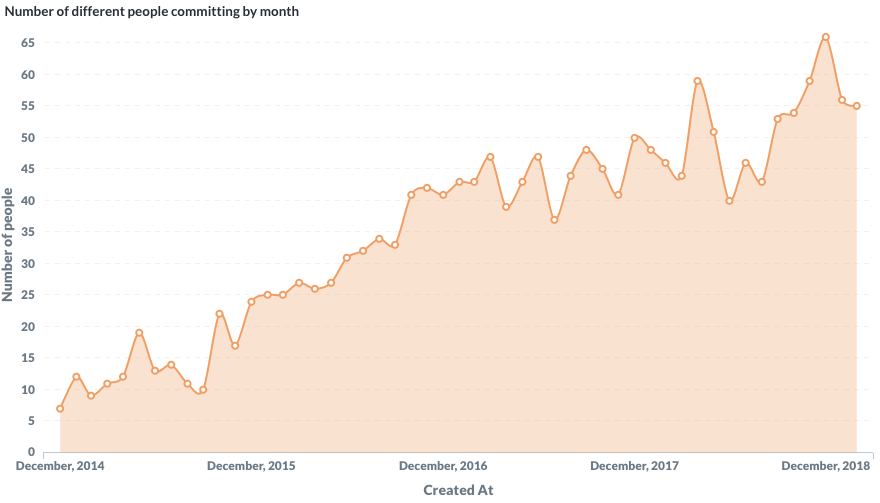
Number of different people writing commits by month
When do people commit during the week? It was funny to spot a slight downward trend from Monday to Friday. Some people push commits during the weekend but 10x less than during the workweek.
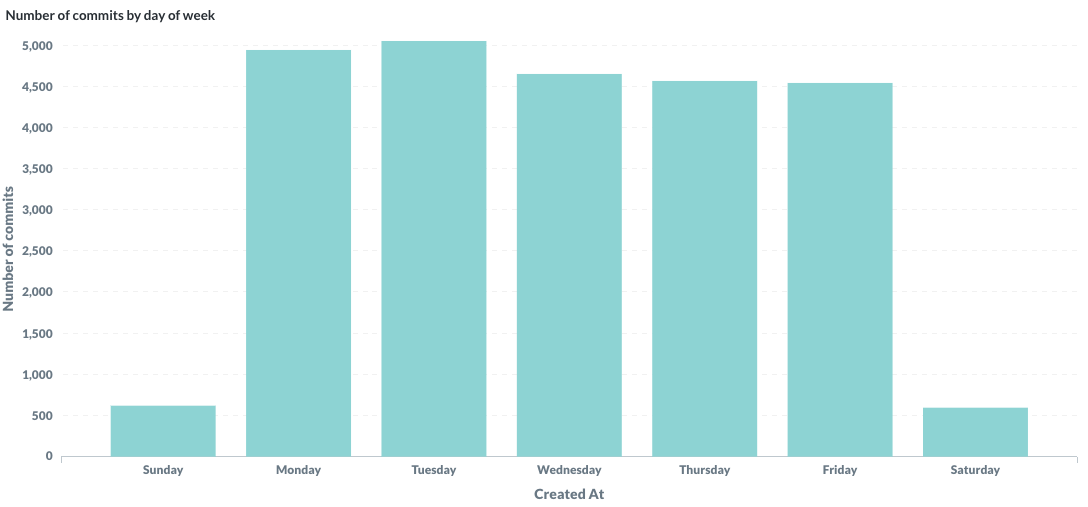
Number of commits by day of week
What time of the day do people push commits? The hour is plotted in UTC but Metropolitan France is UTC+1 or UTC+2. I’m glad to notice a sharp drop at lunch time around noon / 1pm. Eating is super important in France ?.
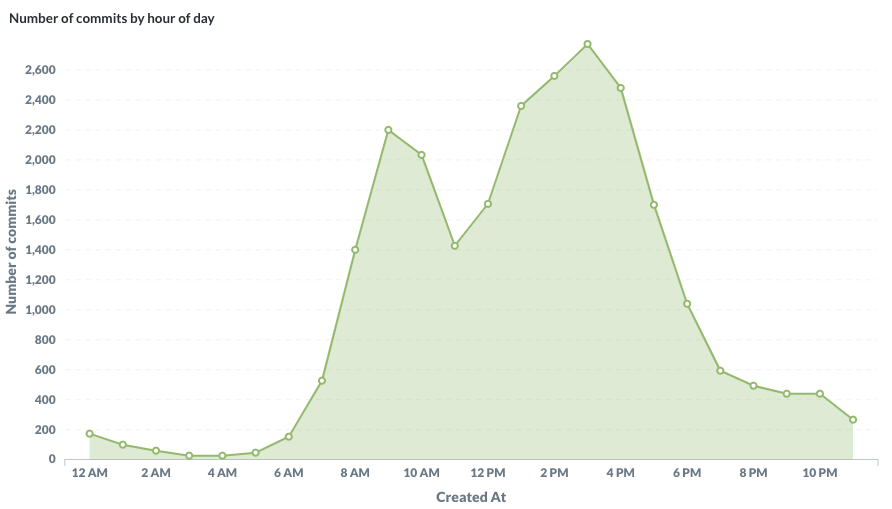
Number of commits by hour (UTC timezone)
What’s the most common commit messages? I bet on something around wip / fix / Initial commit but I was a bit surprised. To spot people who’re doing a lot of commits, I added a second column with the unique number of authors. We can clearly see people who’re using GitHub’s UI to do a commit: Update README.md or Add files via upload.
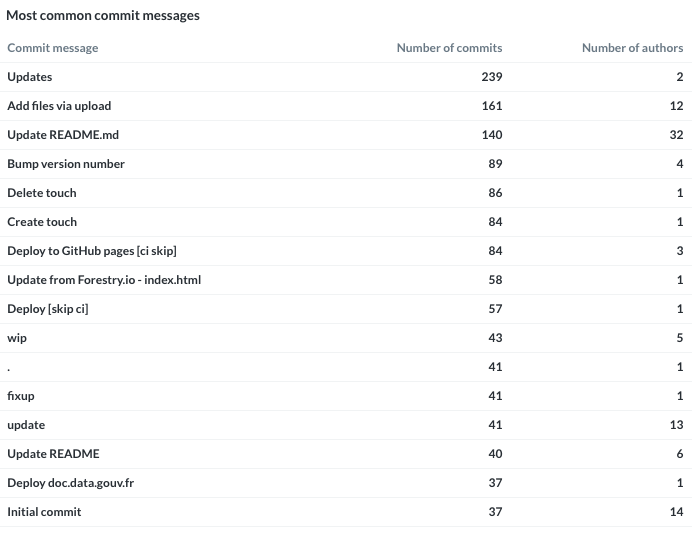
Most common commit messages and associated number of authors
Then, I’ve looked at commit repartition by domain name. I know, it’s far away from the reality but I still had to make a table. I kept only major email domains, defined by the total number of commits recorded.
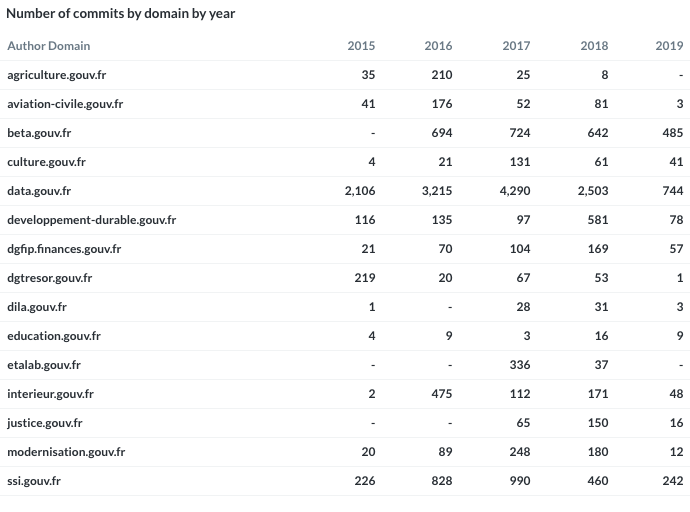
Number of commits by domain by year
Last but not least, I’ve looked at the total number of commits done in a GitHub organisation and the number of unique committers who’ve contributed in this organisation. The first one is the blank organisation: when people contribute to their github.com/[username] or github.com/[someone] where username and someone are individual users, not organisations.
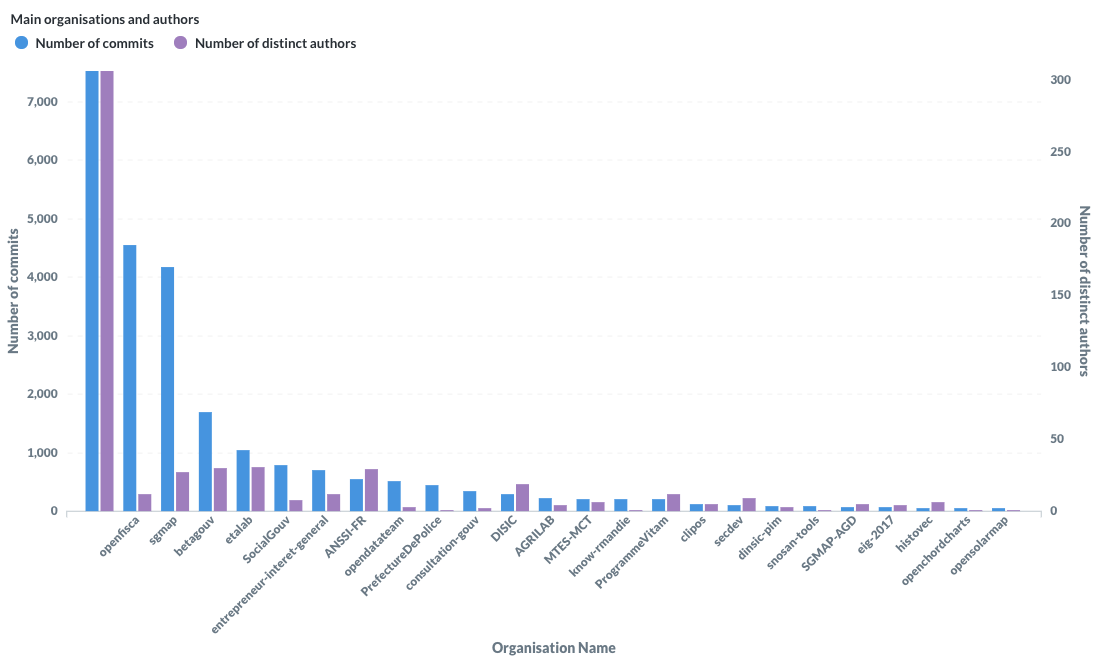
Organisations with highest number of commits
Finally, I’ve looked at contributions to open source projects which are not published by government. I was glad to found contributions to organisations like mozilla, opendnssec, sagemath, krb5, hibernate, legilibre, qgis, rstudio, TheHive-Project or openstack (in no particular order). The French government open-source contribution policy lets people contribute to open source code during work hours, with their own name and using their government email address. I hope it will be easier to collect these contributions in the future.
Doing the same
The code I wrote to extract the data and the dataset itself is available on GitHub and on data.gouv.fr with open source licences. I used standard UNIX programs (wget / gunzip / jq), Python and Metabase. If you feel like doing something like this yourself or using the data, feel free to do so! I’d love to hear about it.
What’s next
This is a personal project but I hope it’s just the beginning. I know how frustrating it is to have numbers so low to show for now and how far from the reality this is. I wrote in a previous article (in French) about how I built a list of repositories and organisations where the public administration publishes code. I’m very interested by these topics so I will continue to work on them and write about it.
In order to move forwards, a few things:
- If you’re working for the French government, use your work email address when publishing or contributing to open source code. Learn how to set your email address in Git;
- If you’re a public administration, register where you publish your code in this file;
- If you’re writing or contributing to open source software, first thanks a lot, and please continue to do so;
- If you’ve got comments, questions or ideas, send an email to
[email protected]
.
I’d like to thank my colleagues Bastien Guerry and Laurent Joubert.