La SNCF est l’entreprise ferroviaire publique française. La filiale « voyageurs » de la SNCF, SNCF Mobilités, transporte plus de 4 millions de personnes tous les jours dans pas moins de 15 000 trains. Une écrasante majorité des voyageurs emprunte des « trains du quotidien ». Ainsi, sur les 4 millions de voyageurs transportés, 2,7 millions de voyageurs empruntent les Transilien et 1 million de voyageurs empruntent les TER (trains express régionaux).
Dans cet article, j’aborde la publication des indicateurs de régularité de la SNCF. La régularité permet d’apprécier le respect de la ponctualité du plan de transport prévu. En termes moins savants : « Est-ce que mon train est arrivé à l’heure ? »
Cet article de blog traite uniquement de la publication des indicateurs de la régularité et non des mesures prises par la SNCF pour améliorer la régularité. La SNCF est engagée au quotidien pour améliorer la régularité des trains circulant ; en témoigne les mesures mises en oeuvre pour améliorer la ponctualité des trains au départ ou l’identification de trains présentant une irrégularité chronique (page 44 du rapport de la Cour des comptes « Les transports express régionaux à l’heure de l’ouverture à la concurrence » du 23 octobre 2019).
Vous avez un train à prendre ? Voici le jeu de données de la régularité de la SNCF à la journée.
Une diffusion de la régularité incomplète
J’ai déjà eu l’occasion d’aborder le sujet de la démarche open data de la SNCF précédemment. Un de mes grands regrets concerne la faible diffusion des éléments de la régularité. Actuellement, la régularité est publiée selon un triplet mois, région, axe au sein de 4 jeux de données à l’échelle nationale. On peut ainsi avoir l’information qu’en septembre 2019, la ligne RER A affiche une ponctualité de 87,6 %. C’est une première information, toutefois, ceci ne correspond pas entièrement à l’attente des usagers. En effet, les usagers des « trains du quotidien » sont intéressés par la ponctualité du lundi au vendredi et durant les heures de pointe, pour ceux qui ont des horaires de bureau.
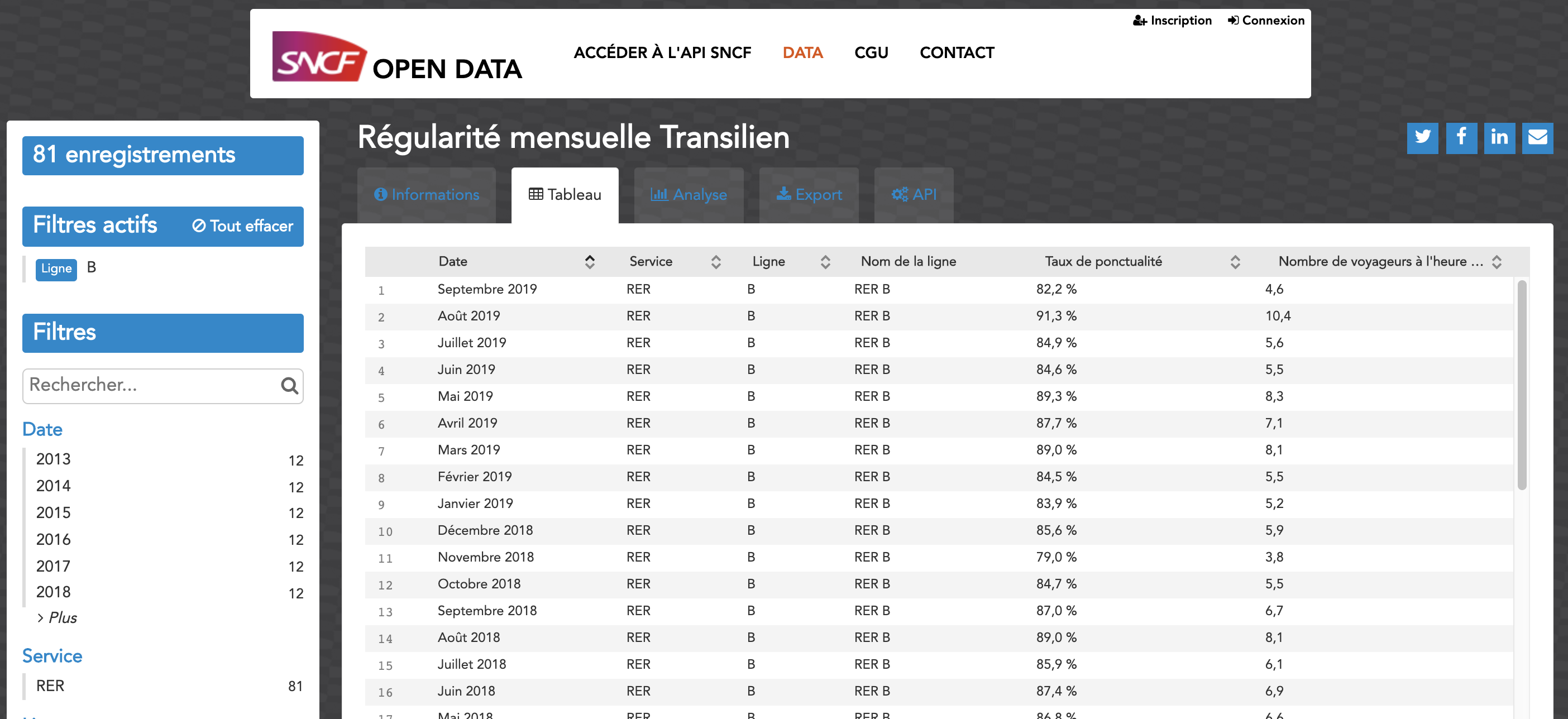
Capture d’écran du site data.sncf.com. Régularité mensuelle de la ligne RER B.
En mettant à disposition des indicateurs mensuels et par ligne, la régularité en jours ouvrés et en pointes est noyée au milieu de la régularité en heures creuses et durant les week-ends (malgré ce défaut, le calcul de la régularité des Transilien pondère sur le nombre de voyageurs, et permet donc une bonne représentativité de la moyenne faite à l’échelle du mois). Au-delà de ce seul exemple, chaque usager devrait avoir les moyens de calculer ou d’accéder à la régularité des transports qu’il utilise. Au minimum, avoir à disposition un indicateur représentatif de sa réalité d’usage des transports ferroviaires.
D’autres initiatives diffusant des informations de régularité
Ma ponctualité Transilien
La branche Transilien de la SNCF propose sur le site maponctualite.transilien.com une information de la régularité plus fine. En effet, on peut obtenir une donnée de régularité après avoir choisi :
- une ligne du réseau Transilien ;
- une gare de départ ;
- une gare d’arrivée ;
- une plage horaire (parmi 4 choix) ;
- une date.
On obtient ensuite un indicateur unique pour la situation choisie. Il n’est pas possible d’obtenir des données sur plusieurs jours (la régularité entre 2 gares, sur la pointe du matin, en semaine, sur un trimestre par exemple). L’export (ou la publication) des données sous-jacentes n’est pas possible, en violation des obligations introduites par la Loi pour une République numérique.
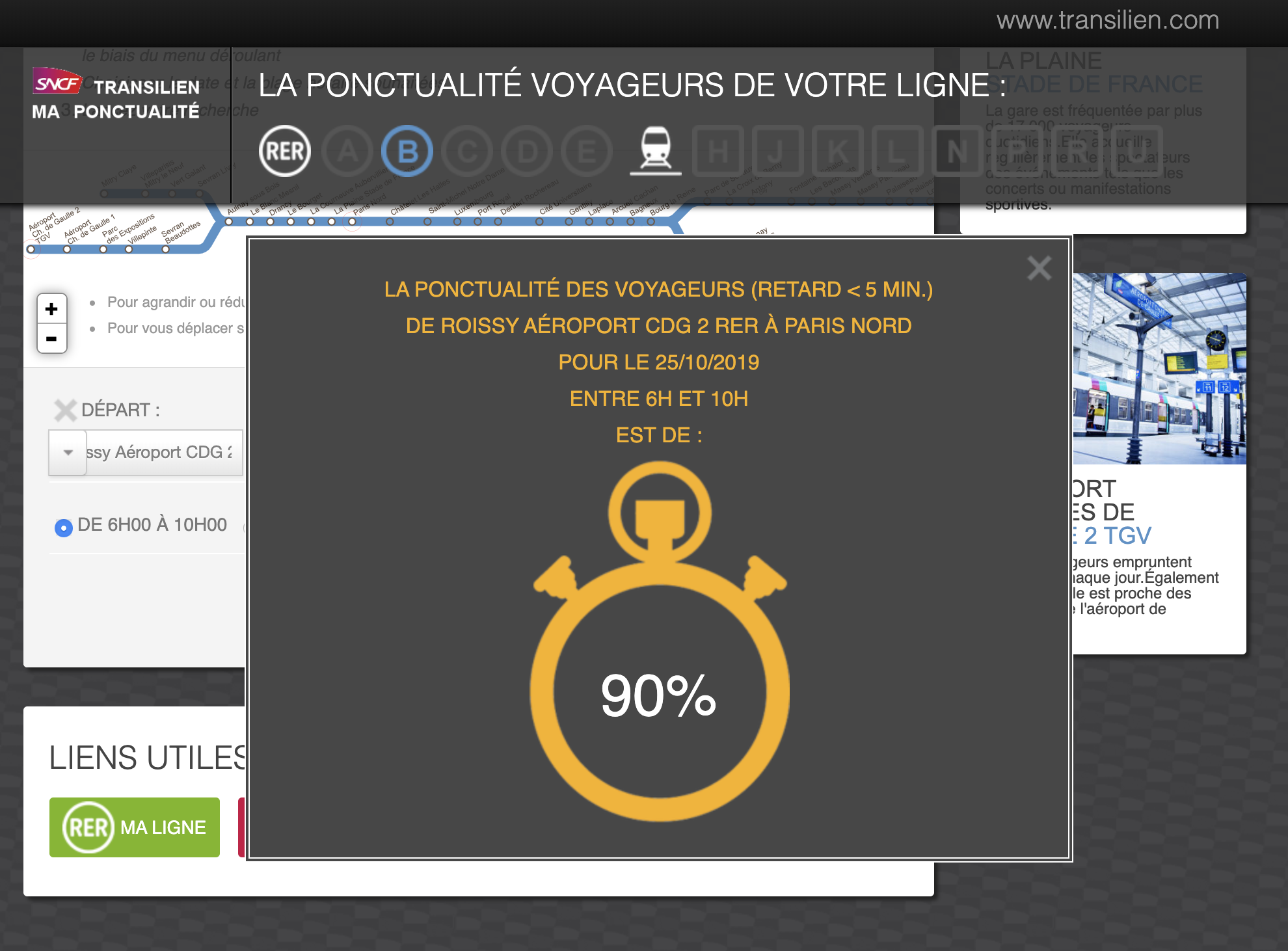
Capture d’écran du site maponctualite.transilien.com. Ce site permet d’obtenir la ponctualité entre 2 gares d’une ligne, sur une tranche horaire prédéfinie et pour une journée donnée. Les données sous-jacentes ne sont pas proposées au téléchargement.
L’Autorité de la qualité de service dans les transports
En rédigeant cet article, j’ai découvert un service du Ministère des Transports : l’autorité de la qualité de service dans les transports (AQST). Ce service a la mission suivante :
contribuer à l’amélioration de la qualité de service dans les transports de voyageurs terrestres (ferroviaires et routiers, urbains et interurbains), maritimes et aériens, en accordant une attention particulière à la régularité, à la ponctualité et à la qualité de l’information diffusée aux voyageurs.
J’espérais trouver des informations inédites de régularité. En parcourant le site web, j’ai pu constater que les données de régularité diffusées pour le ferroviaire étaient identiques aux données open data de la SNCF pour les différentes familles de transport. On retrouve donc au mieux des indicateurs mensuels et par ligne.
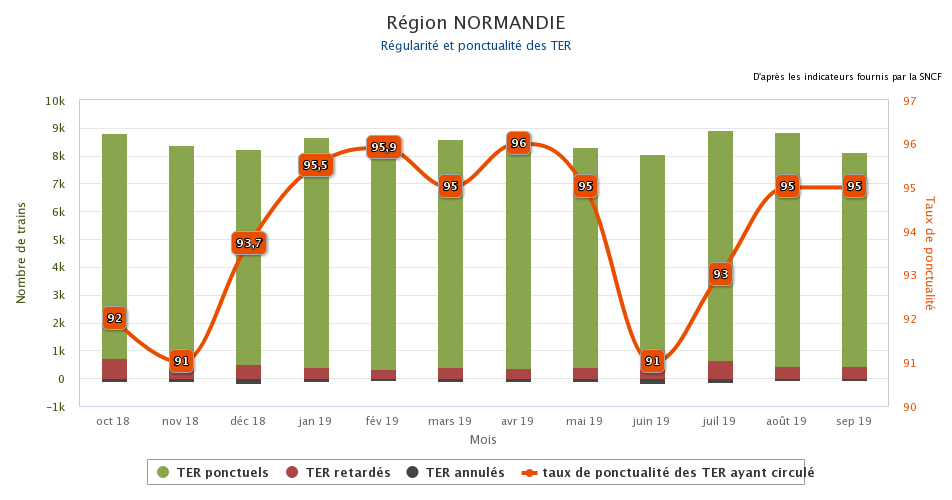
Capture d’écran du site web de l’AQST. On y trouve la régularité des TER en région Normandie sur les 12 derniers mois. Ces données sont identiques aux indicateurs mensuels publiés par la SNCF en open data.
Plus de données ?
Je m’étonne de ne pas voir plus de données publiées par l’AQST ou l’autorité de régulation des transports. En tant qu’autorité de régulation et avec l’aide de la Loi pour une République numérique, ces informations peuvent être obtenues des opérateurs de transport et publiées en open data, sans restrictions d’utilisation. Les choses devraient changer dans les années à venir : le décret n°2019-851 du 20 août 2019 relatif aux informations portant sur les services publics de transport ferroviaire de voyageurs liste les éléments que les autorités organisatrices peuvent obtenir des entreprises fournissant des services de transport au public.
La régularité des trains de la veille : mes trains d’hier
La SNCF propose depuis quelques mois un rapport quotidien sur la régularité des différentes lignes et axes sur le jour précédent. Cette application se nomme Mes trains d’hier. En complément de la régularité, les faits majeurs à l’origine d’une régularité peu satisfaisante sont indiqués.
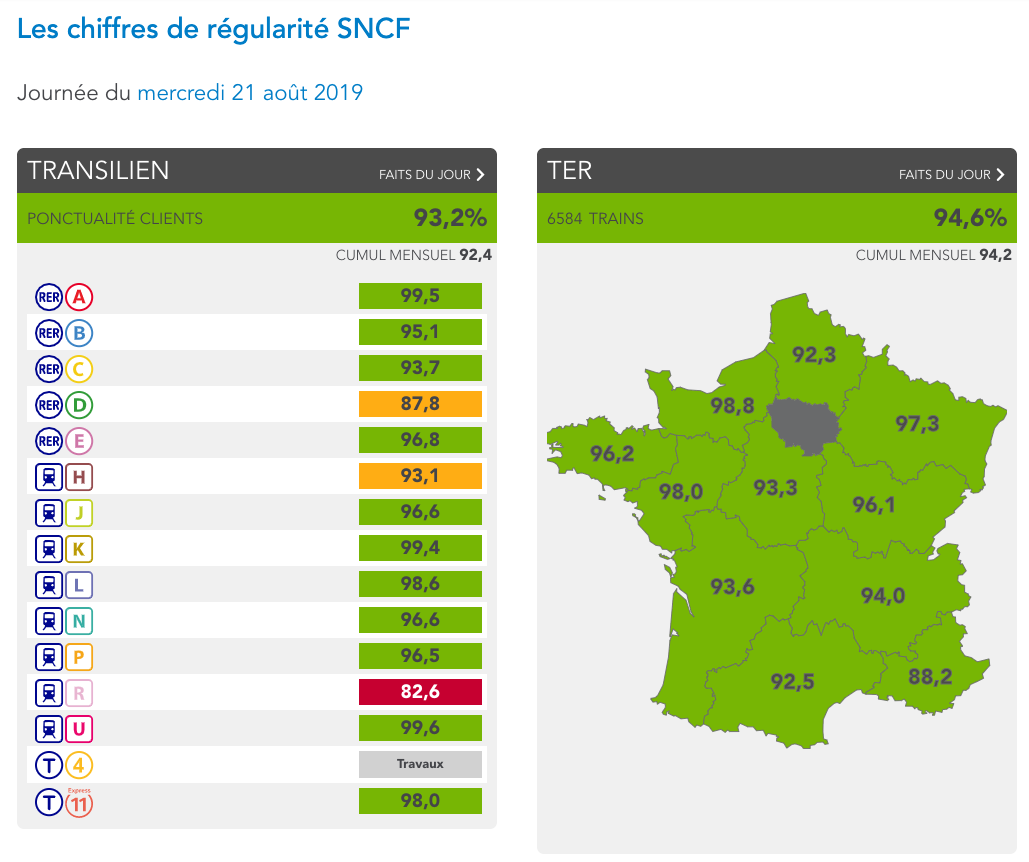
Capture d’écran de l’application Mes trains d’hier. On y retrouve la ponctualité des trains de la veille pour différents modes de transports et par ligne.
Nouveauté notable : les indicateurs de régularité concernent une ligne et une seule journée. En revanche, seules sont affichées les données de la veille. Impossible de choisir une date précédente ou d’exporter des données.
Heureux de voir de telles informations disponibles en ligne, j’ai décidé d’écrire du code sous licence libre visant à récupérer automatiquement ces informations, les structurer et les archiver. Je publie ensuite ces données brutes dans un jeu de données sous licence ouverte.
Ce site web est inspecté automatiquement toutes les heures, car bien que le nom « Mes trains d’hier » suggère que les données affichées soient toujours celles de la veille, en pratique ceci n’est pas respecté. Il est possible que suite à un retard de traitement deux journées soient affichées sur une période de 24 heures. Un traitement toutes les heures permet de ne pas rater des informations potentiellement publiées pendant un court laps de temps.
Je serais heureux que des élus, associations d’usagers, journalistes et citoyens trouvent une utilité à ce jeu de données.
Le ferroviaire, atout majeur de la mobilité en France
Personne n’aime voyager à bord d’un train en retard. Encore moins voir son train supprimé. Le réseau français et la qualité de service globale du pays sont cependant un des meilleurs en Europe et dans le monde, comme le présente ce rapport du BCG. Le secteur ferroviaire français doit être préservé, amélioré et financé dans de justes proportions. Le train est un moyen fiable, efficace pour transporter des centaines de personnes, rapide et écologique. Préservons-le.
PS : cet article a été finalisé à bord d’un TER en région Normandie. Ce train était à l’heure.